Data News — Week 22.36
Data News #22.36 — Arize and Hebbia 💰, Firebolt lay-offs?, data mesh/contracts, dashboard explosion, a big ML Friday and news.
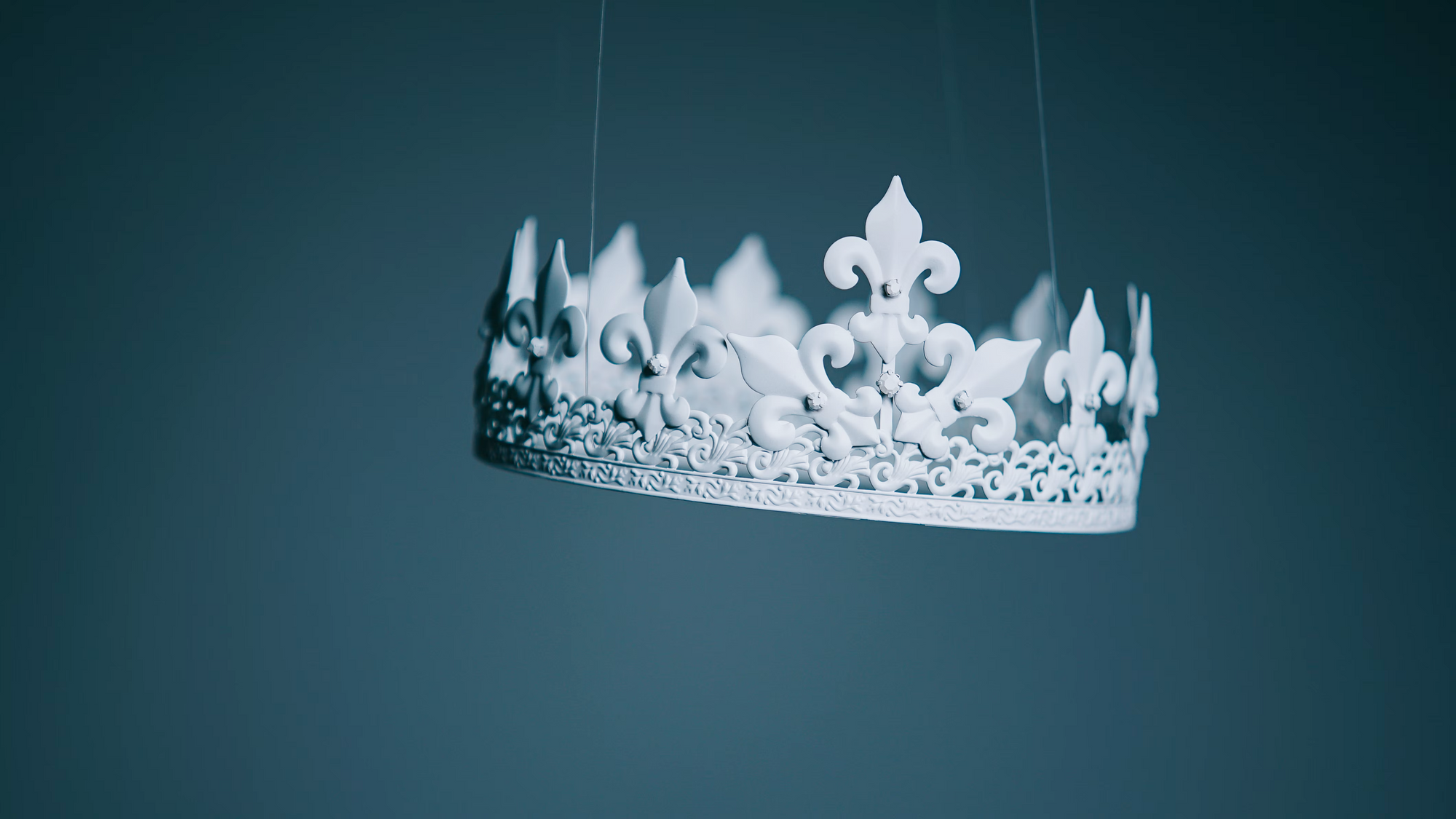
Hey, weeks are passing so fast. Every week I'm like I have time until Friday and here it's already Friday.
On the 21st I'll give a talk in French at a meetup: dbt and the modern data stack. I'll talk about the dbt artifacts and my extension dbt-helper. I'd love to see you there 🤗.
Enjoy this week edition.
Data fundraising 💰
- Arize, a machine learning observability platform, raised $38m Series B. Used by big names. They integrates with the Python standard machine learning stack, with a free tier. If you need drift detection, model monitoring or explainability it's worth looking.
- Hebbia a document search engine raised $30m Series A. Their website does not detail a lot what they are doing and how. You can ingest PDFs, Office docs, etc. and then ask natural language question to get answer.
- 😥 Firebolt is apparently doing a lay-off firing dozen of employees. We don't have more information but if it appears to be true it'll sad. It also shows that the data warehouse competition is harder than ever before and their high valuation — $1.4b in Jan — was a tricky spot to deliver.
- On the same sad note, Snap will shut down Zenly app firing off the whole Paris team. Almost 3 years I was in the same situation with my former employer, I wish all the best to Zenly team. As everyone is saying, Zenly was one of the best French tech team, so if you are looking for talented people try to reach out to them.
Do's and don'ts of data mesh
BlaBlaCar is one of the most advanced French company when it comes to data. The travel company decided to implement a mesh organisation at the beginning of the year rearranging 5 teams into 5 domains. Teams are cross functionnal — like feature teams — in 5 domains: demand, supply (x2), marketing and infrastructure.
In the post Kineret details few do's and don'ts when deciding to move to a mesh structure. As always for a migration the communication is one of the most important topic. With big changes, transparency should come first.
Continuing on the organisation aspect of a mesh, if you want your domain-oriented teams to succeed you'll need to create a way for team to communicate between each other. Data contract is a piece of the puzzle. As data contracts picked up again recently mehdio explained how you can implement data contracts and why it is important.
Small head's up here: you can implement data contracts without an event bus, and even with an event bus you might still need to implement "contracts" that goes deeper than just the messaging system. Because you'll still have exceptions and a lot of stuff will happen outside of the bus.
What if every dashboard self destructed
The title says it all. This is a fun title but it means a lot. In data we have too many things. Many dashboards. Many tables. Many KPIs. What if we automatically destroy dashboards? What if we do it based on views numbers? We could also remove and clean the whole data chain behind a dashboard. In real life I'm not a tidy person, but when it comes to data warehouses or a BI tools I feel this is way more important than my bedroom.
When people are trying to predict the BI future they are often saying that notebooks are the dashboards replacement. I don't think it'll be the case but it's a move forward. In the future of the future people are saying that canvas are the notebooks replacement. I feel this is a good idea, to me it joins the dashboard creativity to the linear execution of the notebook to create a good story.
A small advice I heard this week in the excellent DataGen podcast — Deezer episode, in French. If you use a dashboard, a notebook, a canvas or whatever when you release analysis record an additional video to put sound on your analysis. It will for sure help people onboard faster on your work.
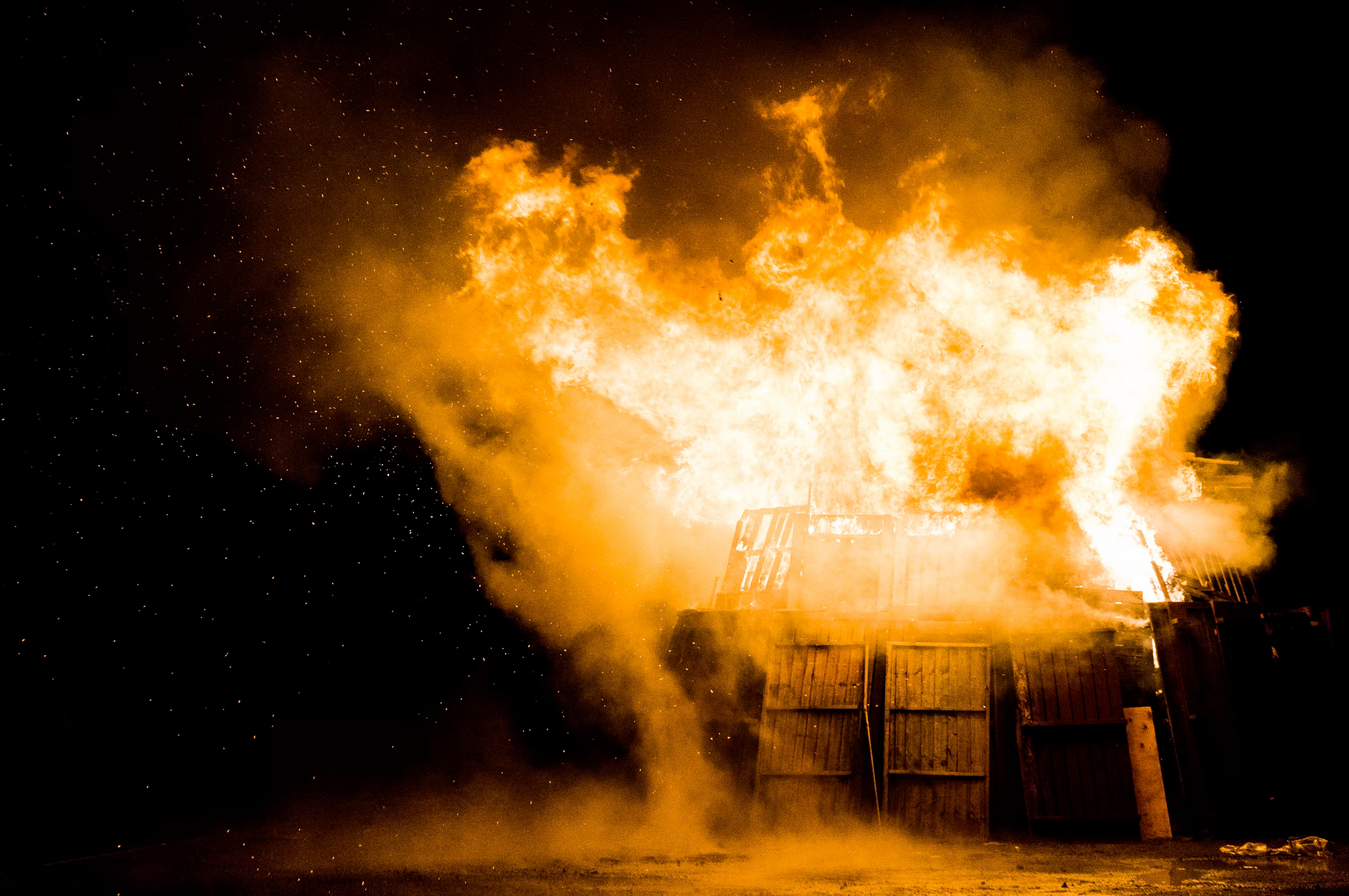
ML Friday
- The journey to real-time machine learning at Instacart — Whatever we way today's data stacks are still mainly batch. The main reason is often because data is used for analytics where batch is enough. That's also why machine learning often starts in batch. But if you want to do production you'll need to be more reactive. Instacart details their journey from batch to real-time with a feature store at the center.
- Unsung saga of MLOps — Jaya from Walmart writes about what are the operational concepts around machine learning in production. Training, modeling and canary deployment in the post.
- Evolving DoorDash’s substitution recommendations algorithm — How a retailer can recommend product when some are not available? This is a great machine learning exercise for aspiring data scientist.
- Recommendations APIs at Slack — This is a bit of an insider post that show where Slack uses ML and also what's the API infrastructure to do it. Mainly batch, orchestrated by Airflow. Next time when slackbot will suggest you to leave a channel you'll know what's behind.
- Recommender System Optimization — Music Tomorrow is a platform that gives knowledge to music professional. They reversed-engineered the Spotify recommendation engine to help music industry create more recommended content ➰.
- Acing the data science interview: 8 practical tips with examples
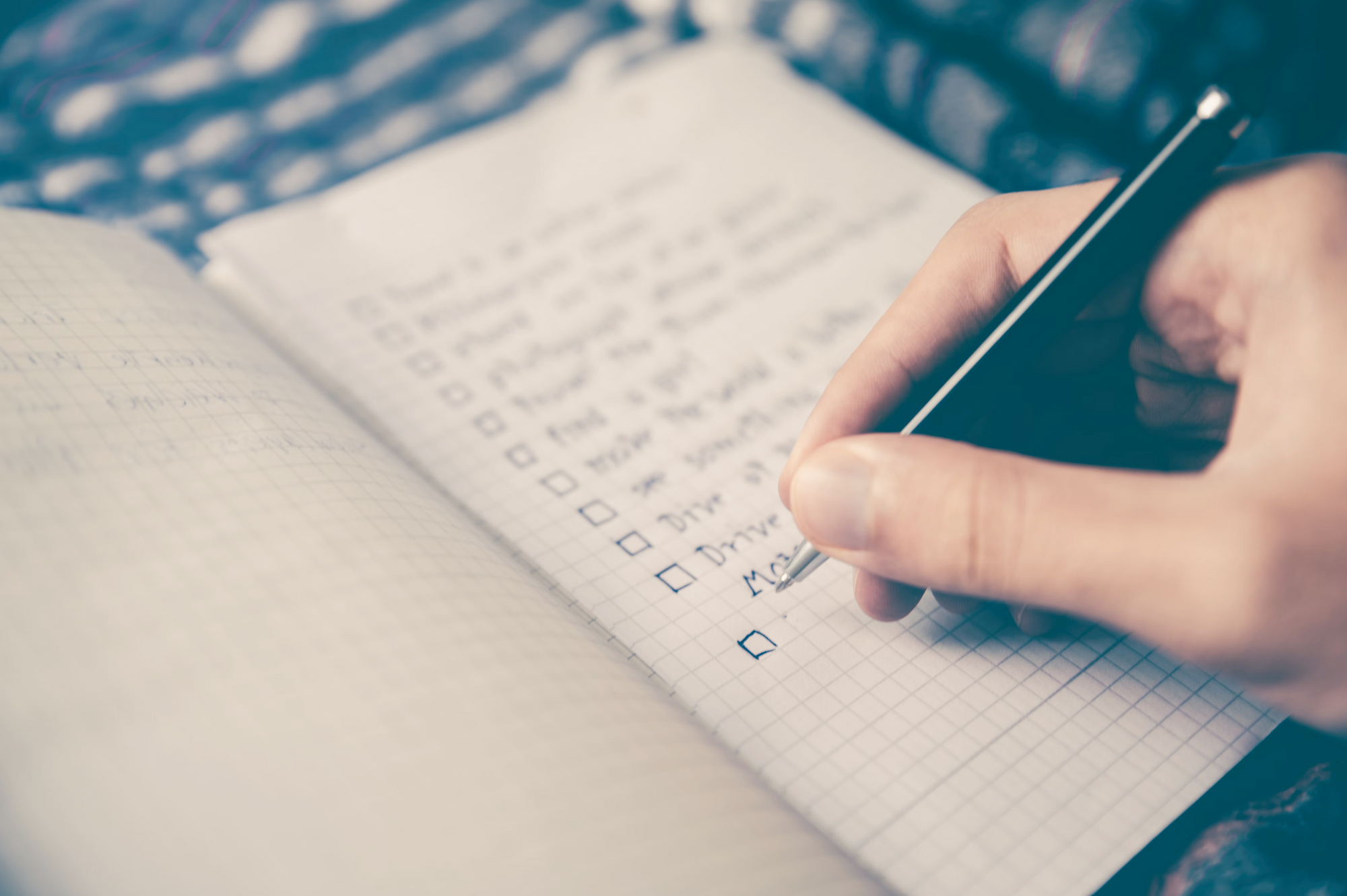
Fast News ⚡️
- ✨ Funnel analysis, a presentation from the Snowflake Summit — If you work in data you have written funnel analysis at least once. Teej made a great presentation on how you can do it in Snowflake. It compares 3 methods joins, windows and regexp and this is clever.
- Parsr — open-source document data extraction toolchain. With Parsr you can clean, parse and extract data from image, pdf, dock and eml.
- Metrics of a data platform — A long list of metrics you can track when running a data platform. If you are just starting do try to implement all at once but do it incrementally. I really like the survey metrics like Ease of getting data and the P90/Time to accommodate. It represents well where a data eng team should perform.
- The pros and cons of Kubernetes — I hate working on Dockerfile and YAML files. This is an infinite loophole.
- Building a Python ecosystem for efficient and reliable development — How Coinbase used Pants to develop a complete build system.
- Why every SaaS company will offer native data pipelines — This is about a trend. I think data connectors is one of the hardest data B2C business to do. So many competitors, so many open-source ways to do it and as it's pipelines, random issues will pop-out every day. Reversing the logic and saying, you have my data so it's up to you to push to me can fix this logic, but tools will need help to do it.
- Terraform 101 — A well written post about what's terraform for beginners.
- How the SQLite virtual machine works — I already spent too much time on this edition, and I did not read this article but I want to.
- Deciding if a data leadership role is something you actually want to do
See you next week.
blef.fr Newsletter
Join the newsletter to receive the latest updates in your inbox.