Data News — Week 22.34
Data News #22.34 — small blog improvements, the explorer beta, SQL optimisations, Hudi, Delta, Iceberg comparison, generative AI.
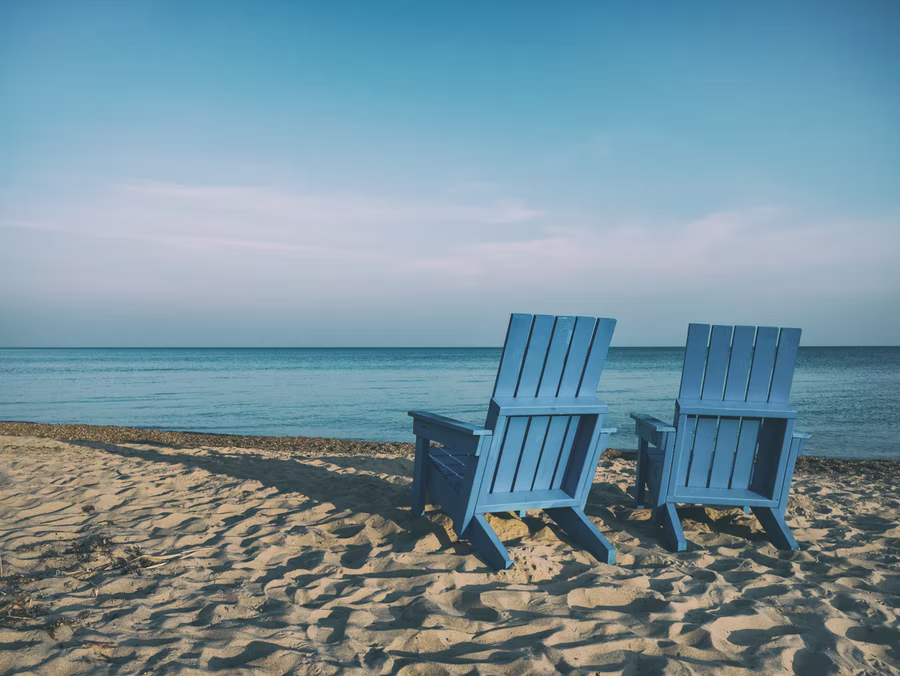
Hey. Already the end of August. Go back to school is approaching. This is a feeling that never left me growing up. You know when you see the summer holidays coming to an end while the stress of the new year is coming.
Who's starting a new work soon? Would you like more content on this topic?
Regarding the blog hygiene, we are slowly approaching the 1400 members. This week I've done a small style refresh of the blog (changing the main color mainly) and the Ghost update so you got 2 cool new features:
- search bar — top right in the navigation bar. This search allows you to search over the posts titles and tl;dr. It's ok but could be better.
- comment system — if you're a member you can comment on all posts.
This week I have also release the the Data Explorer private api. The Explorer will be your hub to search over more than 1000+ data links I've shared in the Data News. With bookmarking, full text search and recommendations. Ping me, I'll give you beta access. Right now I just need to finish the content categorisation.
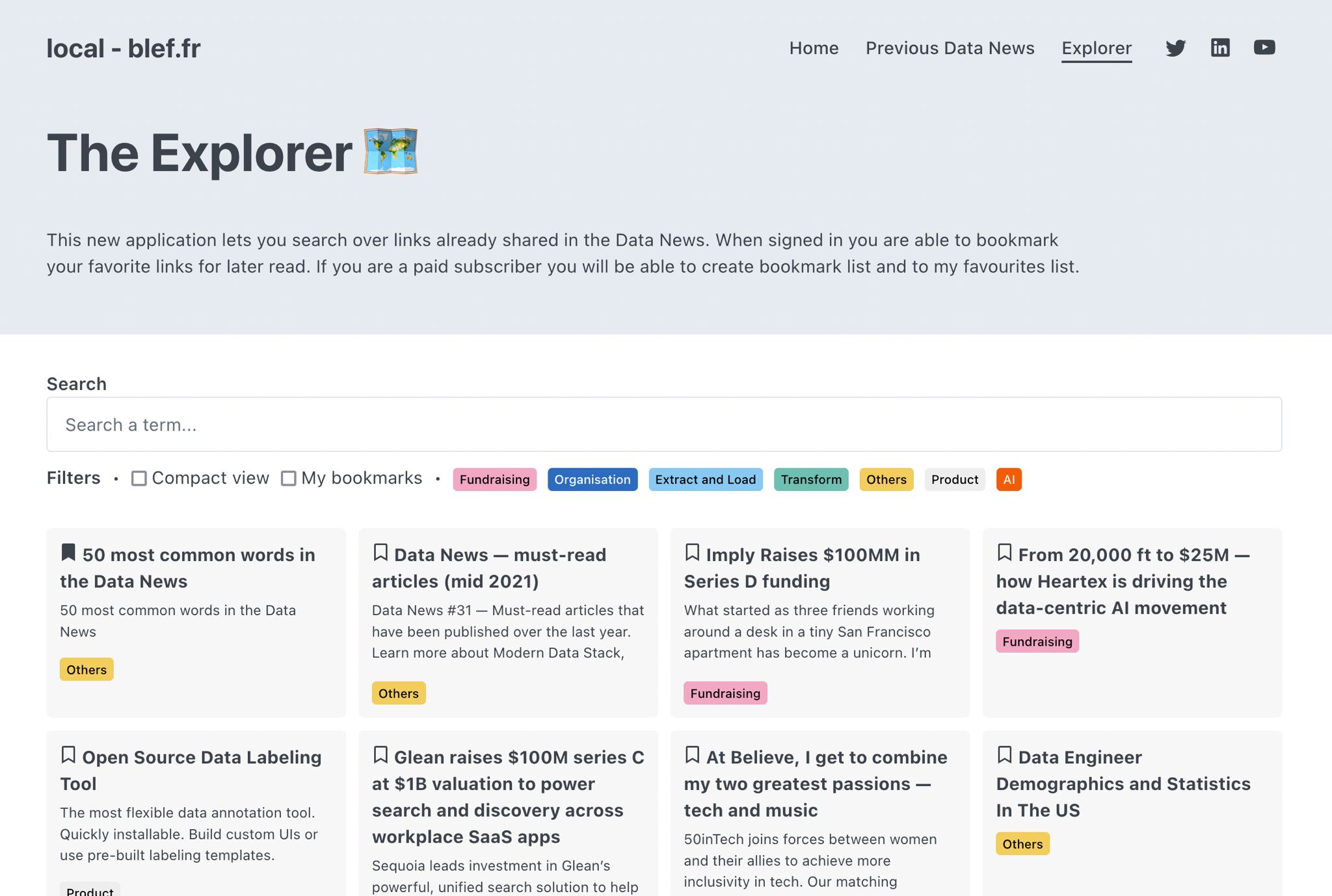
Data fundraising 💰
- The automatic personal data discovery field is booming. Privado, raised $17.5m in funding + Series A to develop a complete suite of tools to monitor all your flows to detect personal data. As I already mentioned in the past, data protections laws are mandatory but tech is not yet ready. I hope these tools will help, but once again the solution is not only technological.
- Qloo raised a $15m Series B. Qloo is an API serving what they call a Cultural AI. It means their AI answers text questions about global trends in the world, like "What books people watching reality shows are reading?". Qloo claims having a consumer datasets with more than 575 entities and being privacy first.
- Zilliz announced $60m Series B to expand their vector database cloud hosting service using Milvus. In a nutshell a vector database is an optimised way to store embedding vectors to compute vector similarity search. These vectors are a modern way to store DL or ML unstructured data once refined.
- Snowflake is on track to buy Applica, an AI based document automation platform. Here the strategy is clear, Snowflake wants to become your all-in-one data store whether it's transactional or analytical. As long as it's data 🤷.
SQL optimisations
Everyone knows that migrating a dbt model to incremental can save you time and money, but we are often lazy to do it. In this article dbt data team explains how they saved $1800/month by migrating to incremental. On the same topic, Péter shows why generated window functions in dbt can lead to degraded performances. It can save $340 per query!
Data products/contracts
The rise of Data Contracts — I've always been a huge fan of the schema registry concept (yes to me it's the same). I think companies should first try to fix their schema management before adding any tool in their stack. Schema registry done correctly fixes everything. But it may be one of the hardest thing to do. It requires a collaboration between tech and data and force SE teams to like databases schema.
Once you have the contracts/schema you can start thinking in term of products/domains.
Hudi vs Delta vs Iceberg — The definitive comparison?
I think I may have shared at least 3 posts in the past regarding the comparison between these 3 technologies. This is probably the last time because this one is really exhaustive. Onehouse compared Hudi, Delta and Iceberg on what they do in term of R/W features, table commodities and platform support. They also explain some key concepts of table storage.
Their opinion should be treated cautiously because Onehouse sell a platform powered by Hudi, so I feel they might be biased at least when it comes to platform support.
To balance opinions, there is a post written by James (Product at Snowflake) on why Apache Iceberg will rule data in the cloud. And another one written by Vladimir on how you can use Delta with Spark.
You might be still lost after reading these two post. My personal advice as someone who never tried the 3: pick one, do stuff with it, learn a lot while using it. Once you become better at identifying what you need, challenge the initial choice.
ML Friday 🤖
This week we have a ML Friday of a decent size. I really like this category because even if I technically understand 10% of what I share I feel attached to it.
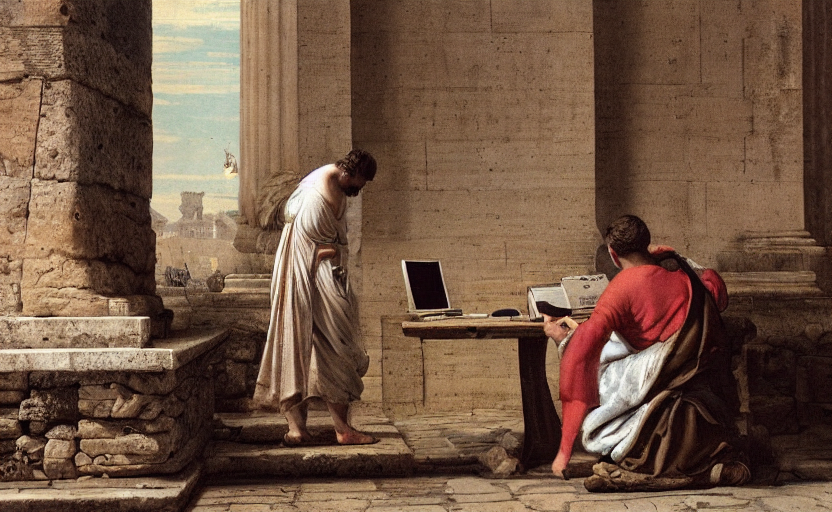
As an appetizer let's chat a bit about generative AI. I really like what these AI are all doing — DALL-E, Midjourney, dreamstudio, Imagen — have built impressing stuff that may change creative process for ever. What will be the future of journalism if we can generate unique images per article? Will artists use AI to avoid the blank page syndrome?
Does it mean we'll live an AI Art Apocalypse in the next years? The author of the article covers very well the topic: economics, why the art, AI as a tool. As in every revolution jobs will be transformed, or worse, lost and we should have empathy for these people doing jobs that may disappear. On the same generative level Google opened a wait list for their experimental AI chatbot.
Other articles are:
- How Instacart uses machine learning-driven autocomplete to help people fill their carts — This is a search x recommendation topic really interesting.
- Causal Forecasting at Lyft, part 2 — Spoiler they predict the weather to guess how many rides they will do. To be honest this is a good decomposition about how it's hard to model a complete business to make forecasts.
- Machine learning streaming with Kafka, Debezium, and BentoML — How to do a real-time recommender system.
- ❤️ Simplicity is an advantage but sadly complexity sells better — Applied to deep learning recommendations models, but can apply to everything and points are really true.
Fast News ⚡️
- 🎓 Learn how to design systems at scale — This is a huge course about system design. It covers a lot of topic in a nice format.
- Observable SQL schema browser — the JavaScript oriented notebook initially dedicated for visualisation is more and more leaning towards data. They released a in-notebook schema browser.
- Managing our data using BigQuery, dbt and Github Actions — This week I went to a Google Group meetup, one of the presenter said that Cloud Build is awesome. It's a cheap container execution engine that works. Github Actions is also close to be the same.
- Spark tips, Optimizing JDBC data source reads — A great dive into levers you can use to optimize db reads.
- Migrating Databricks tasks from Prefect 1 to Prefect 2 — Prefect released recently the v2, this post shows you what are the key difference on an example.
- Professional Pandas: the Pandas assign method and chaining — From a Pandas master. Learn how to better use assign (I should read this post more often because I don't like assign).
- What's the big deal about key-value databases like FoundationDB and RocksDB? — This is the last link of a already too long newsletter. Read it if you find the title catchy, personally I like it.
See you later 👋
blef.fr Newsletter
Join the newsletter to receive the latest updates in your inbox.