Data News — Week 22.21
Data News #22.21 — Observability fundraising, Preql & Actiondesk, Manage your data resources, what is Pinot?, Modern Data Stack for charity, etc.
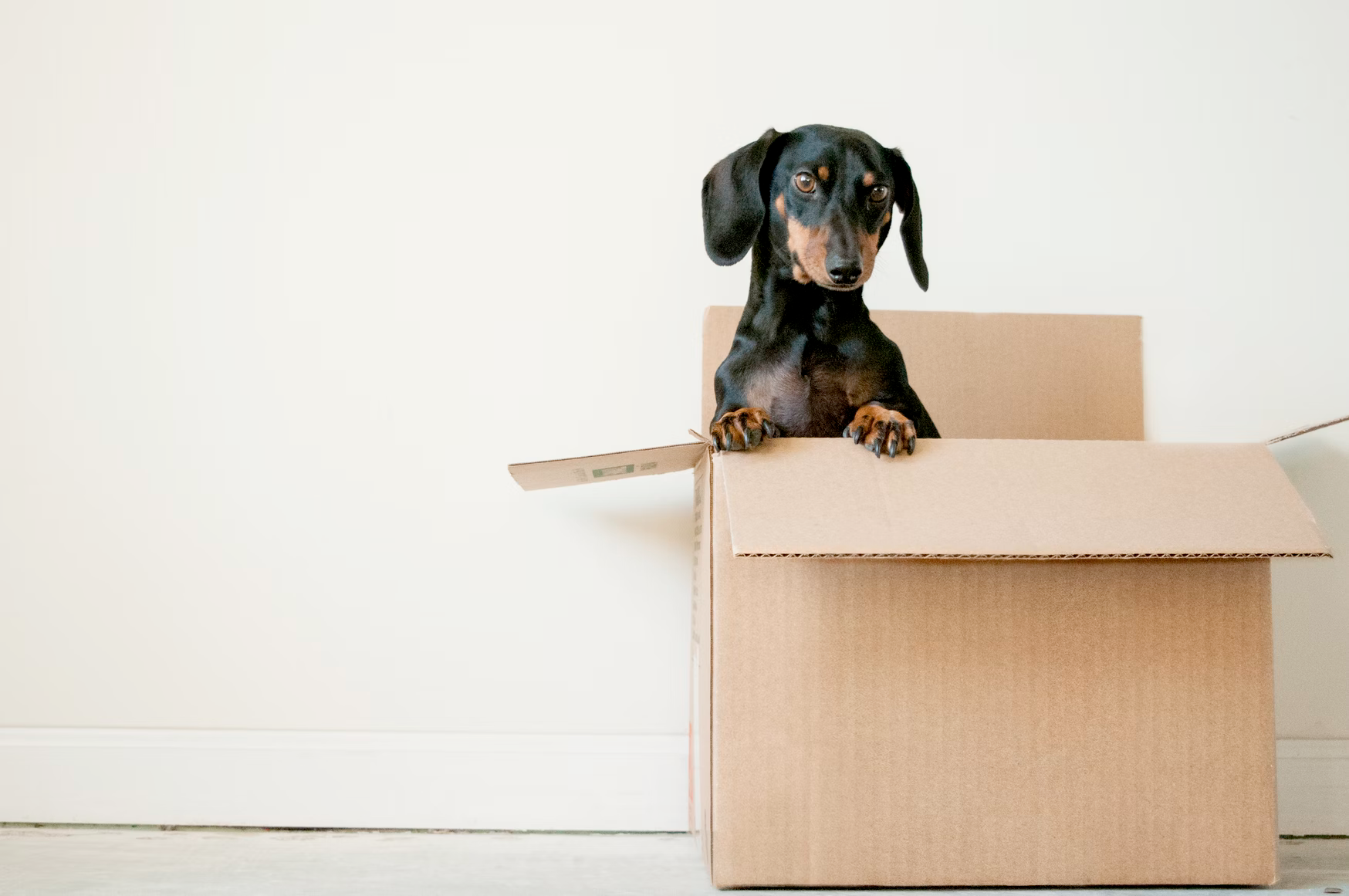
Hello everyone. I hope this email finds you well. Back to the usual Data News. While the Airflow Summit is taking place I also plan to do a takeaway post after watching all the replays.
For the next two Fridays I'll be in holidays ☀️ but I'll try to plan 2 posts in advance. Have a good read.
Data fundraising 💰
After some slowness in the fundraising in the last months due the global economical situation this week is bringing some cash in the data space.
- The data observability space is heating up, this week 3 companies raised money on this specific topic. Monte Carlo, one of the most known and influent data observability company, raised $135m Series D1. MC is pioneering the field with a lot of content and probably a great product — but I haven't tried it yet. At the same time Cribl got $150M in Series D funding to do stuff I did not understand. They have a product called Crible Edge which is a observability agent. Finally Manta, a data lineage company, raised $35m in Series B to expand their lineage technology to solve observability issues.
- River, a graphical SaaS ETL, raised $30m in Series B. The usual boring promise of a all-in-one tool to do everything about data, the easy way.
- Something more exciting, Preql raised in funding $7m to build an intelligent transformation layer to speed-up your analytics workflow. "No data team or SQL required" as the landing page is stating.
- Actiondesk raised $3.9m to build a data warehouse as a spreadsheet solution. They connect to your databases or apps and with templates create directly business reports in a spreadsheet like interface.
- Broadcom to acquire VMware in a $61B deal. With the cloud, the virtualization still exists and a lot of companies still relies on these technologies. A small wake-up call.
PS: the stuff around observability is weird, it feels like companies are raising on these keywords just for the money. It probably says something about current trends. Feel free to correct me if I'm wrong.
Manage your data resources
You know I'm fan of data organization articles. I really like to share these kind of articles because as of today this is super hard to create data teams that works. There are a lot of considerations and Emily says that you should not run your data team like a product team, you should run it like a company that needs to scale.
She recently changed position and put some thoughts around the fact that actually your data team could be run like a product team, but not only. And she details this "not only" part. Data job is different. In the post she asks really good question you should ask yourself to build the best data team.
In parallel Tristan from dbt Labs took Emily's post as a starting point and elaborated on what you can do to avoid velocity traps for your team. In summary for Tristan software engineering job is divided between releasing user features and everything else. Deciding how to allocate your team's time between both is probably the hardest part. When it comes to data it's a bit different but not that fat. This is the data hierarchy of needs.
To be honest I recommended you to read the two original posts I'm not sure my takeaways honour them 🙈.
As a side note here another aspect to reduce your team costs: stop useless workloads and services.
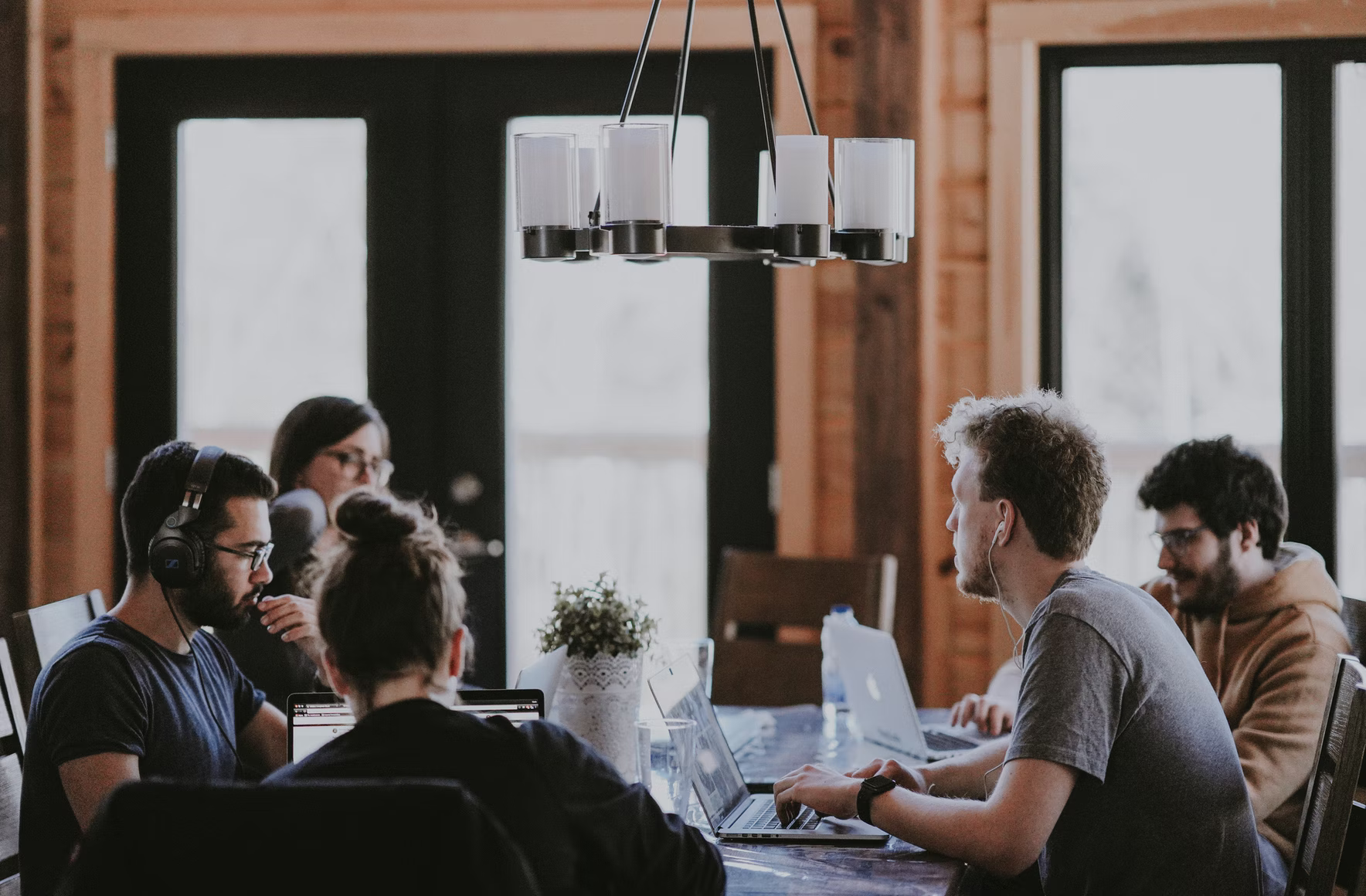
Lessons learned from running Apache Airflow at Scale
While waiting for other Airflow insights from the Summit here a first feedback. Shopify lists lessons learned running Airflow 2.2 with more than 10k DAGs. They noticed that the multi-tenancy is not perfect because, author privileges are too broad and this is hard to split DAG ownership. Below an extract of the conclusion.
To sum up [their] key takeaways:
• A combination of GCS and NFS allows for both performant and easy to use file management.
• Metadata retention policies can reduce degradation of Airflow performance.
• A centralized metadata repository can be used to track DAG origins and ownership.
• DAG Policies are great for enforcing standards and limitations on jobs.
• Standardized schedule generation can reduce or eliminate bursts in traffic.
• Airflow provides multiple mechanisms for managing resource contention.
How I have set up a cost-effective Modern Data Stack for a charity
In France we have a non-profit association called Data For Good, their work is awesome. People are working for the public good by solving data issues within their capabilities.
This time Marie detailed how she developed a Modern Data Stack for a Paris-based solidarity grocery. To me this is one of the best article regarding data platform development. She greatly explains every choice and propose a state-of-the-art cost effective data stack.
What is Apache Pinot?
In the Apache wine cellar I would like a Pinot.
If you want to understand what is Apache Pinot, this is a great whiteboard YouTube video explaining why Pinot was created and how you can use it today. In summary Pinot is a realtime distributed OLAP datastore to answer realtime data analytics needs. The video vulgarizes key concepts.
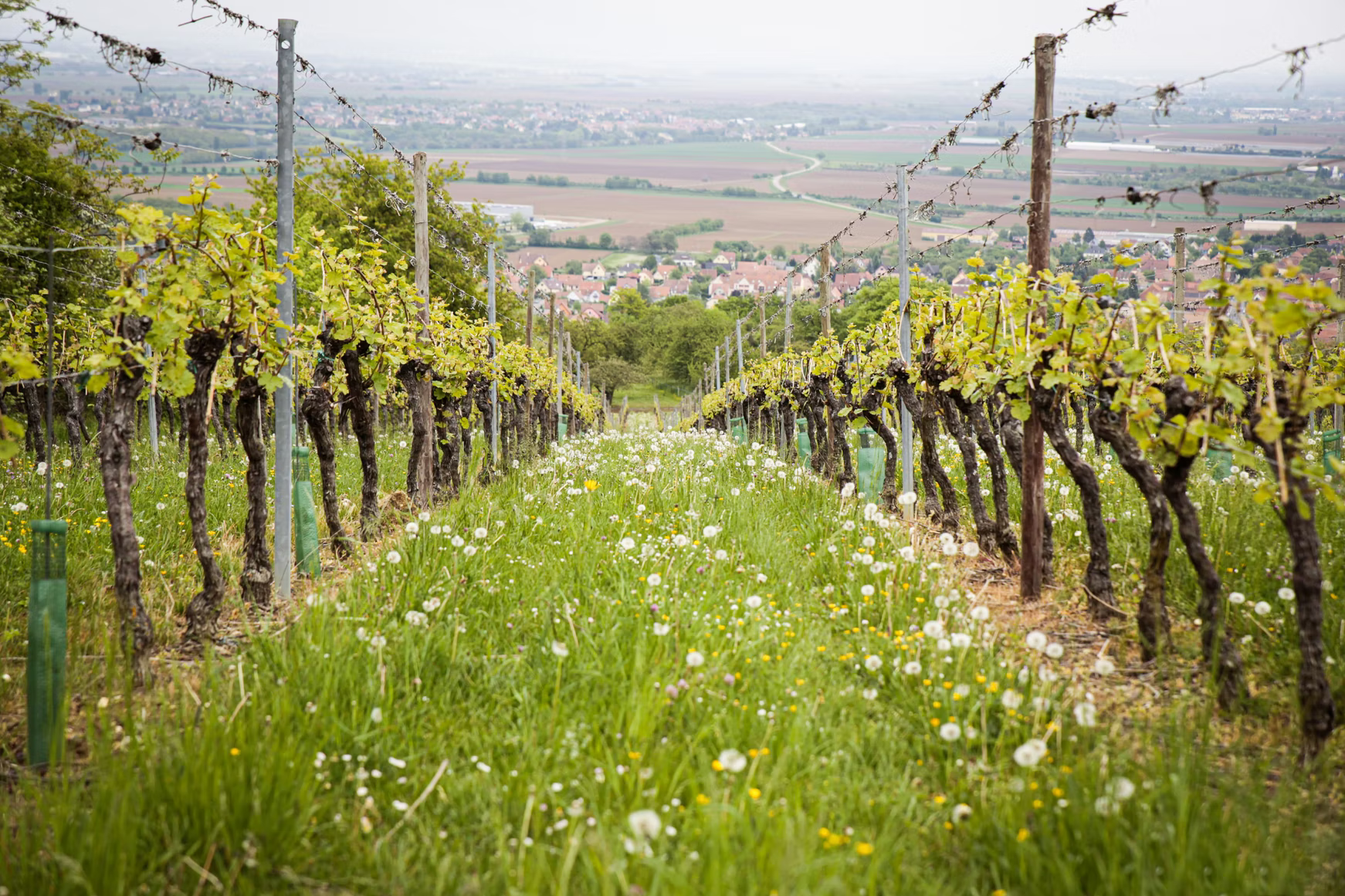
Some product news 📰
- Twitter have been fined $150m because they illegally sold people's data to sell targeted ads
- Amplitude finally released a Customer Data Platform in addition to their product analytics standard feature. Sync up to 10M rows per months and get business metrics and quality checks.
- Integrate PowerBI apps within PowerPoint. Yep, this is not the usual Modern Data Stack stuff, but everyone do some slides at some point and being able to add directly dashboards inside will help PowerBI expansion.
- Apache YuniKorn becomes a Apache Top-Level Project. YuniKorn is a lightweight resources scheduler that sits on top of Kubernetes dedicated for Big Data workloads. The project also includes a UI.
Opinion post: Can ML be absorbed by the DBMS?
George, the CEO of Fivetran, asks if machine learning could be a simple database task? Obviously this is already technically feasible, but will we see a major paradigm change like few years ago when we said "data langage is SQL"? The future is open.
Fast News ⚡️
- How Git truly works — When you work in data being a Git user became mandatory. No matters what you version if you version it. This post explains more how Git managed hashes and versioning.
- How Git can help analytics work — In addition to the technical explanation here a summary of engineering best practices (like versioning) we should apply to analytics.
- Consider better alternatives to CSVs — File formats can change dramatically application performance. When working with data you should consider using Parquet.
- Machine learning model observability — A podcast on how you can approach observability with models and how it's different than monitoring.
- 5 biggest Data Engineering mistakes — Usual reminder on what we should always consider.
- Why Python is more complex than you think — A YouTube video from PyconDE — DE for Germany and not Data Engineering, haha — that shows small funny specificities in Python and why Python will become harder with the time.
- Atlas, manage your database schema from CLI — I discovered this CLI tool to manage your database schema like a Terraform but for databases. Here the recent release post where I discovered it.
- Timely Advice – How Long Does Dataviz Take? — A dataviz visualizing how long dataviz projects are taking 🌀.
1 Monte Carlo's VC, Redpoint, wrote a post congratulating Barr Moses (CEO) vision.
blef.fr Newsletter
Join the newsletter to receive the latest updates in your inbox.