Data News — Week 15
Data News #15 — Union.ai (Flyte) fundraising, Datadogs of tomorrow, Kafka and Presto at Uber, Feathr a new feature store, a lot of fast news.
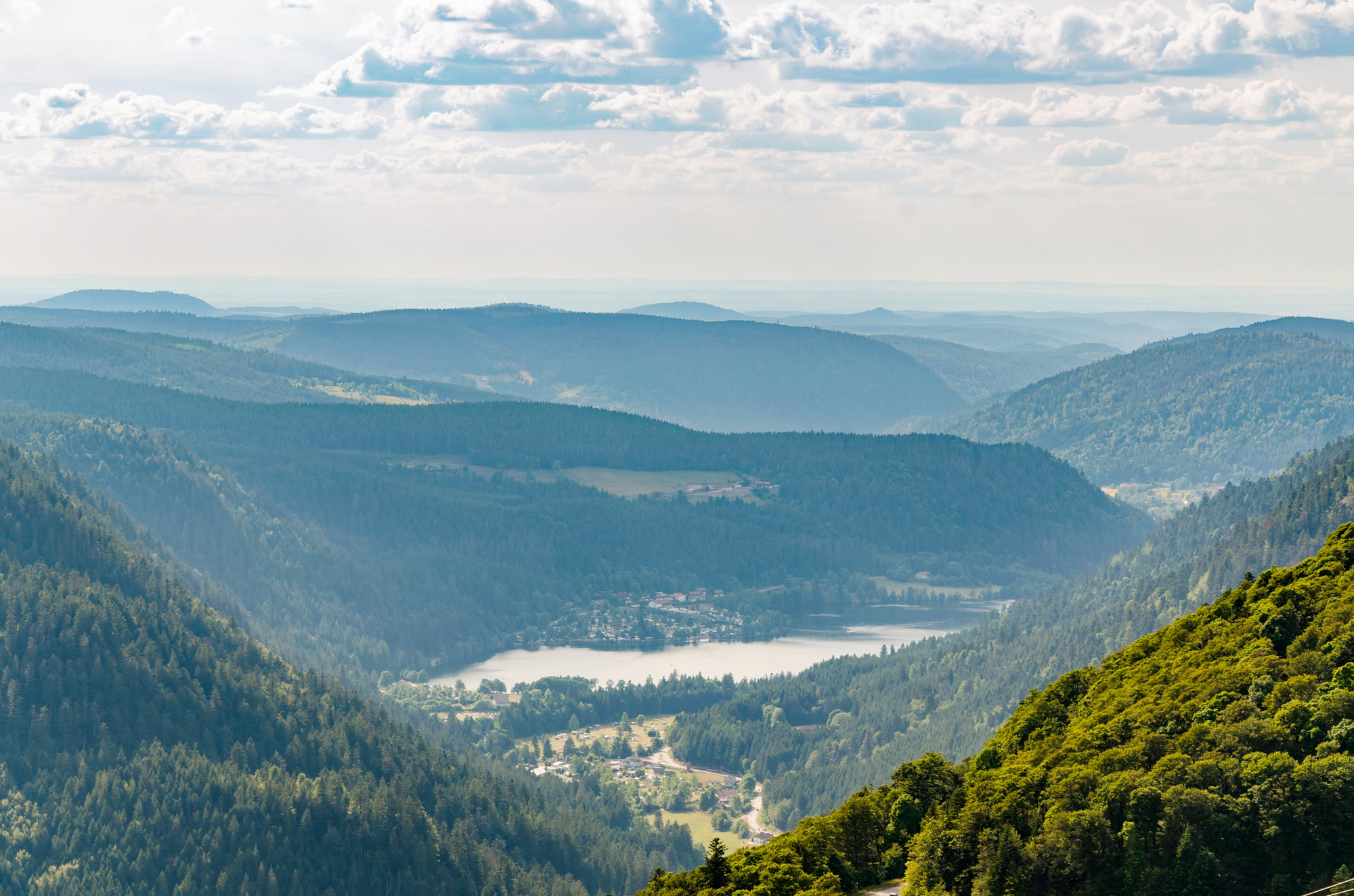
Bonjour Data News readers. In order for me to prepare the anniversary community special edition if you have time could you send me your 3 favourite articles you read recently, but written at anytime. And for fun can you also send me the place where you are when you are reading this newsletter edition — on my side I enjoy the sun in the mountains ☀️.
Data fundraising 💰
Union.ai raised $10m in a seed round for another workflow orchestration tool built on top of Kubernetes. They are the team behind Flyte, the workflow orchestration tool chosen by Spotify to replace Luigi and initially developed at Lyft. This is impressive how the startup soft power today comes from open-source frameworks. Back in the days Luigi lost the battle against Airflow — in the background Airbnb vs. Spotify. And now Spotify is coming back with the round 2. With a lot of money and more competitors.
Then if we look at marketing and how Union.ai position the product in the market we see that they sell a ML and Data Science tool rather than a generic pipeline management system. This is something I've also notice while chatting with Prefect team, companies do not want to face Airflow generic capacities but address Airflow flaws particularly in ML space. Even though the Apache project by its generic nature can cover everything. In the end it's just about writing Python.
As a side note Flyte is written in Go.
The Datadogs of tomorrow
This is clearly the line drawn by data observability tools, they want to become the Datadog of the data field following the success of the company — valued at $50b. Which is a bit ironical because why can't we use the original Datadog rather than a copy?
Data Discovery Tool: why you absolutely need one!
Anas from HiPay shared what made his team pick Amundsen as discovery tool for their data platform. If you are still in the process to find the needs for this kind of tool in your company it'll help you for sure.
Kafka analytics at massive scale at Uber
Uber data teams rely heavily on Kafka when it comes to data infrastructure. In summary they are event driven and everything goes inside. After Kafka a lot of different tools are playing their part. Presto has a big role in this and they operator 15 clusters with 7000 weekly active users. This is massive. They detailed how Presto interacts with Kafka.
If you want an entry-level post Khandelwal explained step by step how you can query Kafka from Presto.
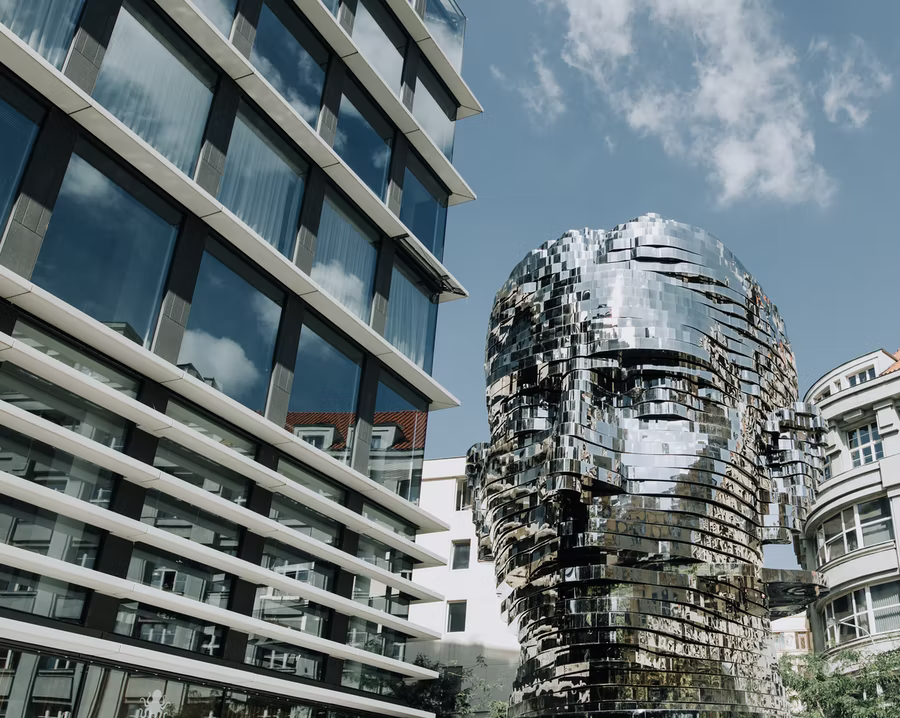
Feathr, a new feature store, entering the game
LinkedIn open-sourced their feature store, Feathr. It is written in Scala. For people not familiar with the matter a feature store is a centralized data store dedicated to machine learning features. The idea behind is to factorize ml features computation and results. Thank to it we can avoid repeating same feature engineering in each micro-service.
Feathr is built out of multiple components: offline store (object and SQL) + online store, a feature registry and compute engine. The online store proposed on Github Readme is Redis.
The second news in the post is that Feathr will also be provided to Azure cloud users.
Three tips to save BigQuery costs with immediate effect
I have to admit that I'm ashamed not knowing the second tip. Montadhar wrote 3 BigQuery tips to save costs. Which means saving query time. Which means in the end saving company money.
Fast News ⚡️
- Snowflake Data Clean Rooms feature — This is something I discovered while doing the curation. Snowflake provide a way to do data sharing while preserving statistical secret to avoid risk of reidentification. Honestly from the article I don't get how it works and I'm more afraid of the tracking use-cases it would enable to avoid privacy protection laws. But yeah, it exists 🤷♂️.
- Last week I shared Google BigLake announcement, Ben summarized Data Cloud Summit in a medium post.
- Create your
requirements.txt
using this technique — I voluntarily kept the original title, but do not use this technique to create your requirements files. Prefer usingpip-tools
orpoetry
. - Use Python Fire package to encapsulate your Airflow tasks (for KubernetesPodOperator) — Avi proposed a pattern to replace PythonOperator by Fire + KubePodOperator. Fire is a auto-cli generation tool from an object.
- LakeFS comparison of Hudi, Iceberg and Delta Lake — A great post to get the vocabulary associated to new table formats.
- Be careful with Jupyter notebooks publicly exposed — This post have been spammed around social networks, but the message is still valid. There are exploits on Jupyter notebooks so be careful when you run a quick and dirty instance on your enterprise cloud.
- Data engineering best practices — Matt wrote several practices to apply when working on data eng projects.
- Data Engineering career path at gov.uk — This is a good ol' post from January 2020, the Crown defined what data engineering is there. This is a way to get inspiration for your job offers or career paths.
- Embedded Analytics vs Data Apps — Firebolt CPO tries to define where data apps domain lands versus embedded analytics. Spoiler alert: it depends on the team that will develop the stuff.
- Factless Fact table — a strange concept where your fact table contains no fact. The concept is fun but I have difficulties imagine this in a enterprise world, this is related to Chad Sanderson views about broken data warehouse.
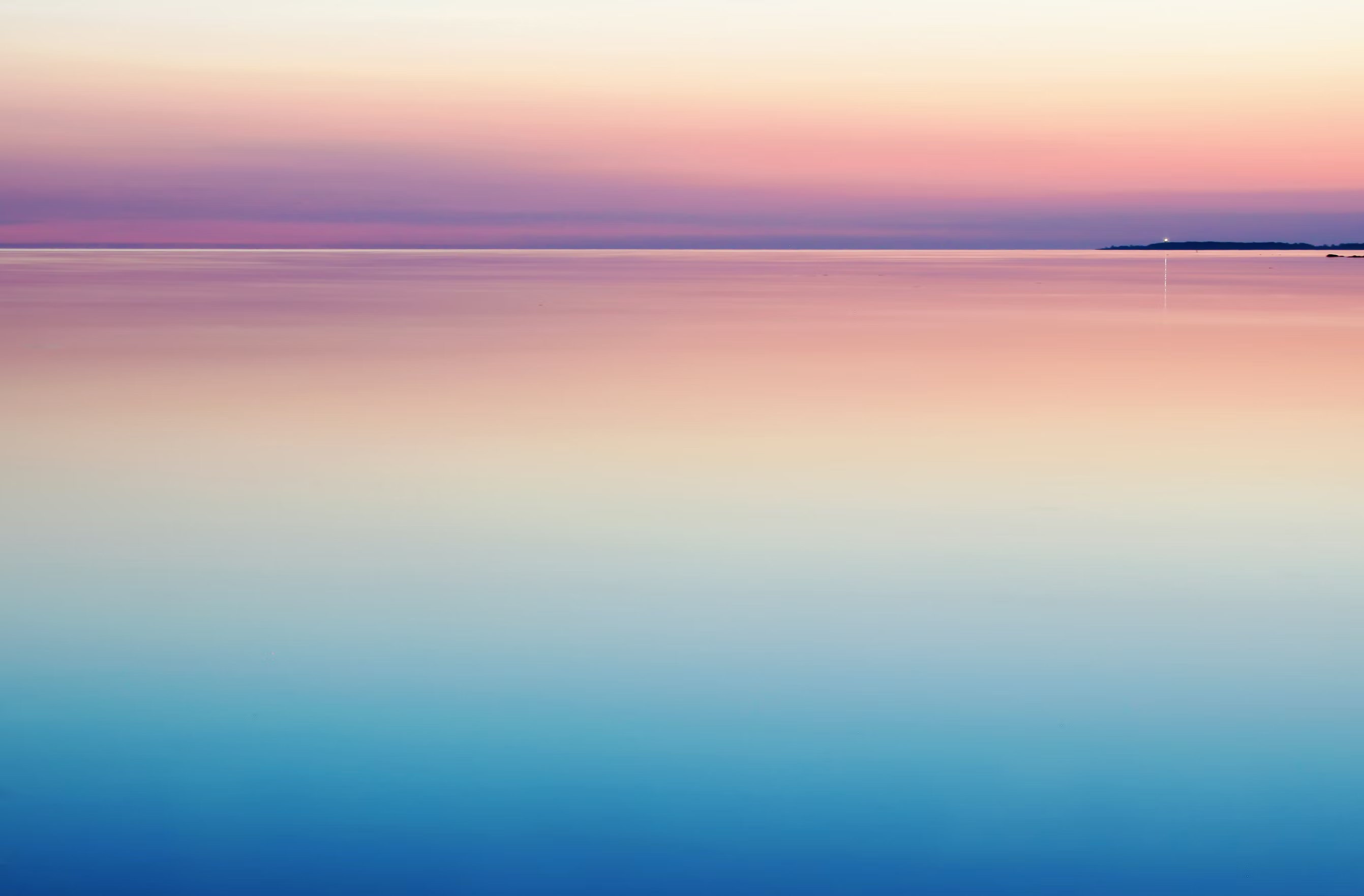
No comments 💬
New category where I just share bare links (and also I have nothing to say but I like the articles).
blef.fr Newsletter
Join the newsletter to receive the latest updates in your inbox.