Data News — Week 10
Data News #10 — Atlan, Canner and DataRails fundraising, data platforms future, 7 antifragile principles for dwh, the art of CLI.
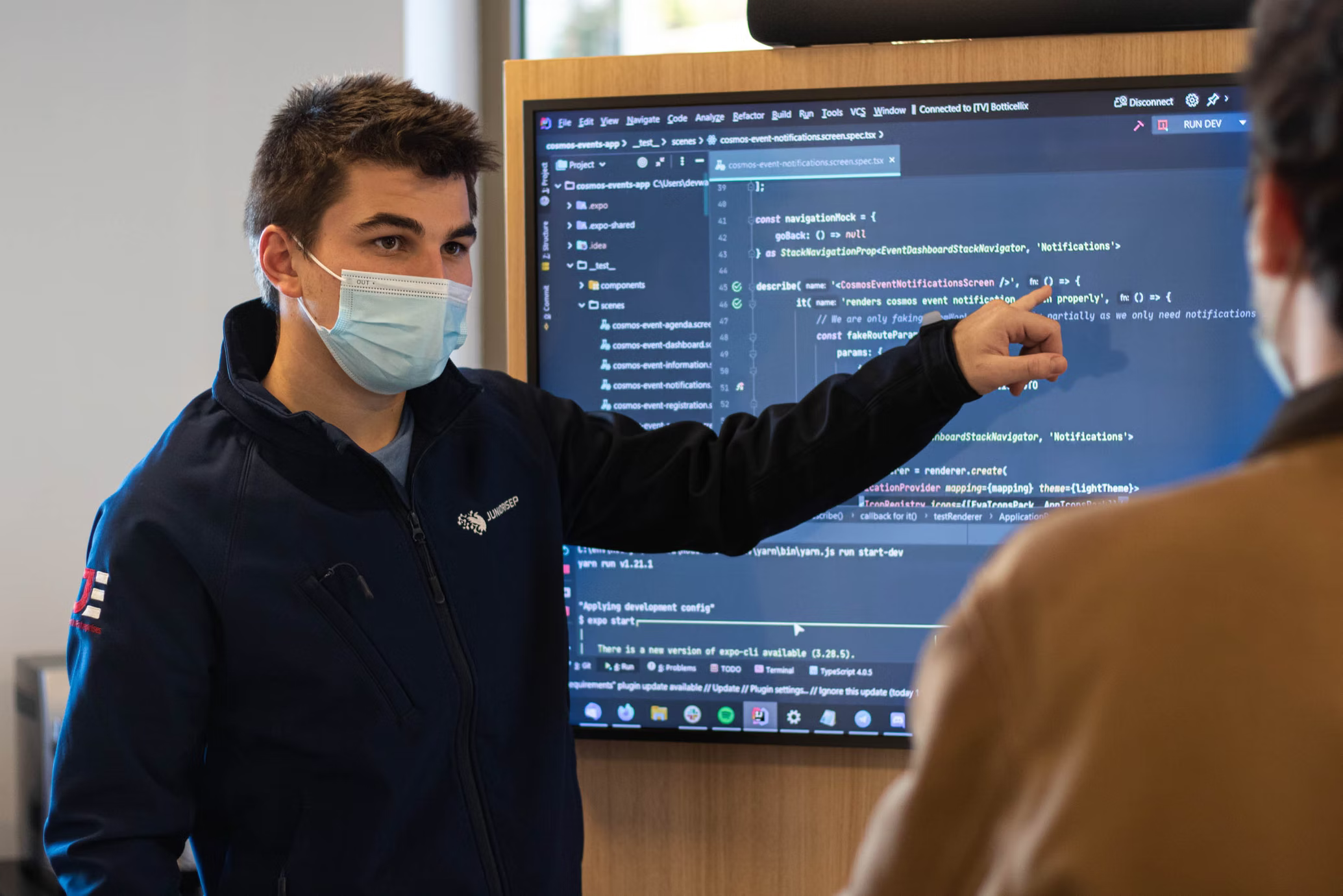
Dear members, I hope this edition finds you well. I'm sorry to be late once again, but it coming to an end. I've been giving classes since January almost every Friday and I've only 2 left in the next weeks so the original schedule will be back soon.
Data fundraising 💰
- Atlan raised $50m in Series B to continue building their home for data teams. Which means in our vocabulary a data catalog-lineage — we should call this datacatalineage. Their vision is to become what Github has become for tech team but for data teams.
- Canner, a Taiwanese startup, raised $3.5m in pre-Series A. Canner provide a data mesh approach on top of your existing tools. Connecting all your data storages, the product provide as an output an universal data access after transformation. This is a meta platform from data storage to data serving delivering data mesh philosophy.
- This week DataRails raised $50m in Series B to provide a financial planning and analytics (FP&A) solution for Excel users. It means they developed in-app plugins to empowers your financial team.
Let's speak about Excel. Excel has been here for years and it has been a saviour for a lot of people and a lot of companies. The Excel ecosystem is huge, maybe wider than the data ecosystem. With all the efforts we all made, Excel is still a reference, people will still want to export your lovely Tableau dashboard into an Excel spreadsheet. We could name this Shadow data in relation to Shadow IT.
Data platforms future
This week Petr and Benn spoke about data platforms, not really from the same perspective but to me they are both saying the same thing: data stacks are built on top of core tools/concepts where everything else should be encapsulated inside at some point.
Petr proposed a different way to bundle data platforms. Or to rephrase it, a different way to categorise data tools. In a nutshell, today we have more than 20 categories of tools in the data ecosystem. This is a big number and we should relabel everything. On top of core layers — ingestion, storage, transformation, visualization and discovery — we need to provide cross-layers features like scheduling, orchestration, etc.
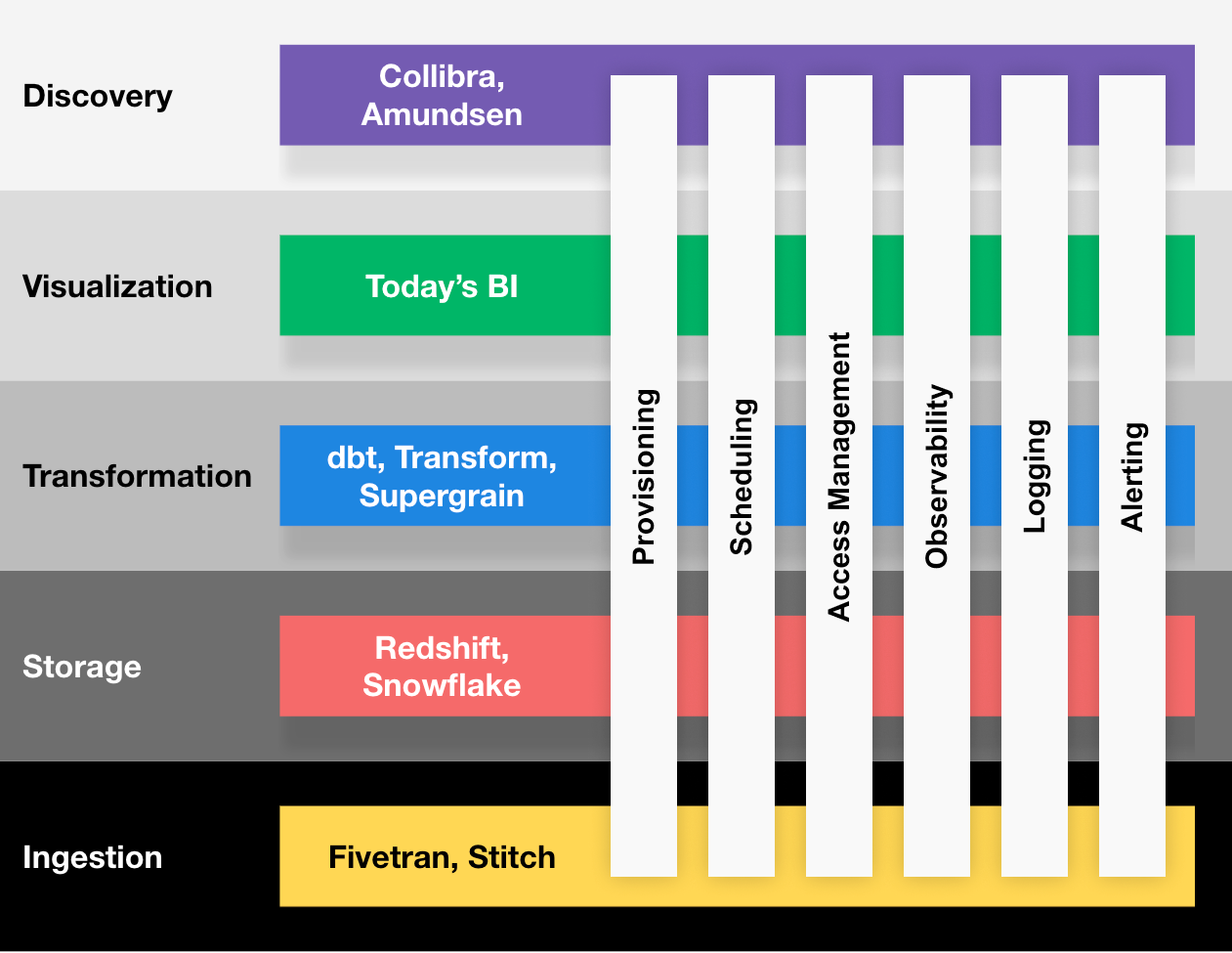
The experience of teams running data platforms depends on their ability to handle the above problems cohesively across the stack. These problems are hard to solve in isolation within each tool.
I really like Petr conclusion of the unbundling Airflow conversation that has started few weeks ago.
On his side Benn followed-up on the very big deal Snowflake put in place last week by acquiring Streamlit. Benn is placing a bet on the data app store concept. Snowflake acquisition could lead to this strategy. If we consider the warehouse like the main piece everything will exist through his marketplace — or his data app store.
I also learn from Benn's post that Google laid off Looker's departement of Customer Love (more detail here). Once again Google strategy with Looker is hard to follow (Week 41 — Google partnership with Tableau), Looker was perfectly positionned in the Modern Data Stack and from the outside it seems they are ripping it off.
7 antifragile principles for a successful data warehouse
Iliana wrote a series of article about data engineering. In the last part (the 5th one) she detailled 7 antifragile principles to build your data warehouse. I think this post is a great ressources to think about the place of your data warehouse and to review processes around.
Source systems are accountable and responsible for resolving data issues
The second principle is stating that source systems are accountable for data issues. Amen. This is so true. But also so difficult to put in place in the real world because product teams hate data migration. You should check also the 6 other principles.
Solving concurrency in event-driven microservices
If you are trying to understand concurrency in event-driven architectures this post is for you. Hugo is also proposing a solution on top of Kafka to deal with concurrency by design rather by implementation.
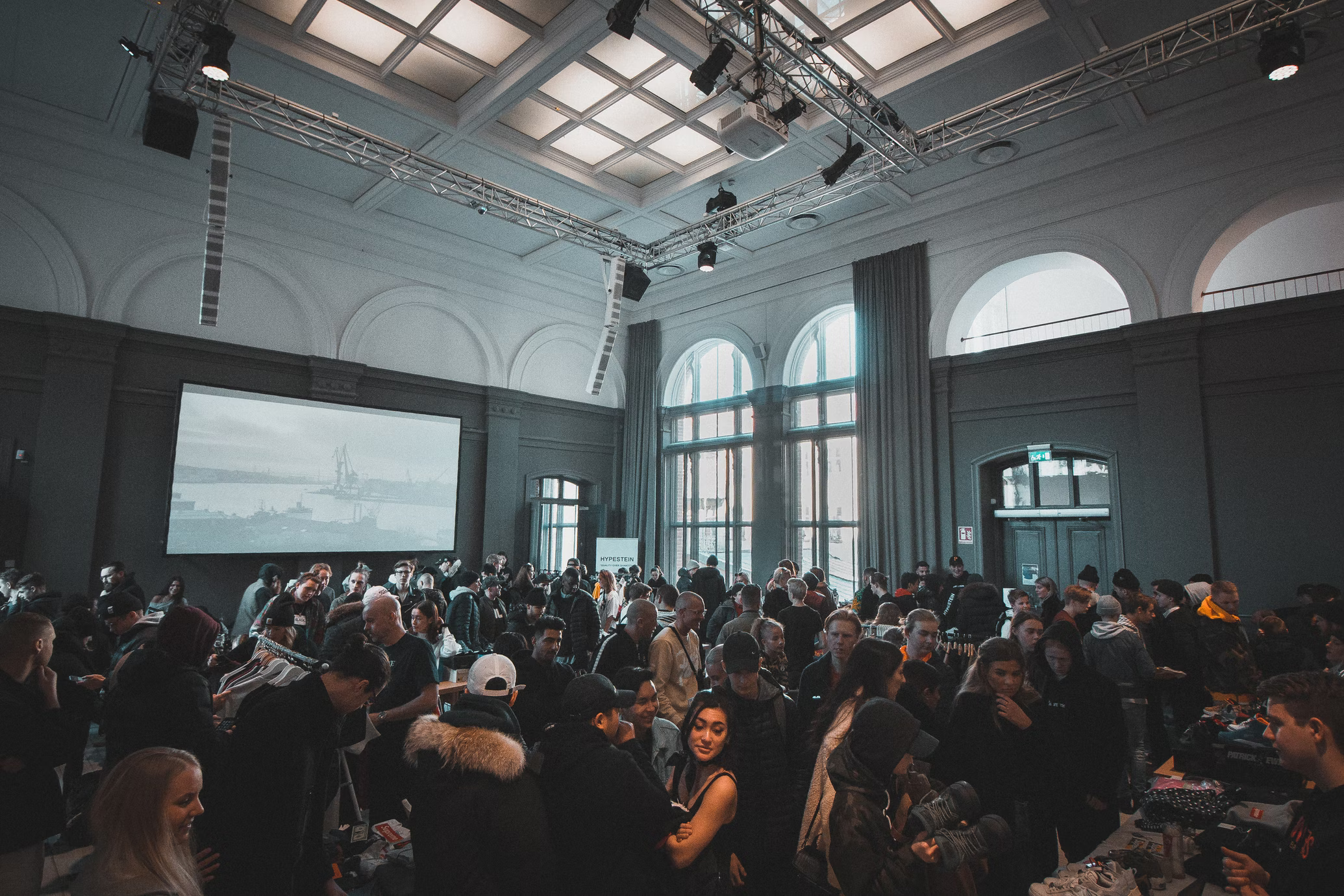
Saturday ML 🦾
Two articles this week in this category. This is food for thoughts.
- MLOps is a mess but that's to be expected — MLOps and DataOps are still in their first days, like we saw earlier in the newsletter data platforms are fragmented and we need a better cross tooling view, we need more cross automation and so on. DevOps should continue to infuse data community to build the best solution.
- Recommendations for all of us — why is it so hard to build recommendation systems for the household? How can Netflix and Spotify be so bad at recommending content for couples that are using the same account? This post tries to explore solutions to this recommendation problem.
Fast News ⚡️
- A gentle introduction to Terraform — For all newcomers to want to understand what are the main layers in terraform code.
- One stone, three birds: finer-grained encryption @ Apache Parquet™ — Uber team detailled how they use Parquet encryption features to support their security controls.
- Mastering pivot tables in dbt — After Furcy pivot in Spark on top of BigQuery, this week we see how to master pivot table in dbt.
- 🥋 The art of command line — Awesome Github README to guide you through the command line, I bet you're gonna learn something from it.
- Parallel grouped aggregation in DuckDB — DuckDB is a database management system designed for OLAP. Thanks to their vectorized query execution engine they achieve awesome performance. In this post they explain how they do parallel grouped aggregation. The post is useful to understand database internals.
- Data Tidier — Convert messy tabular data into a tidy format.
See you next week.
blef.fr Newsletter
Join the newsletter to receive the latest updates in your inbox.